|
"Let’s talk"-Platform
In the digital era, polarized online debates have become rather a rule than an exception. In the framework of our experimental platform “Let’s talk” (developed with the help of CDLX Berlin) we tried to create additional incentives for online discussants to engage in democratic listening. To this end, in our experiment, we developed a set of listening-oriented reaction buttons designed to signal gratefulness, respect, persistent disagreement, the feeling of being heard, and curiosity.
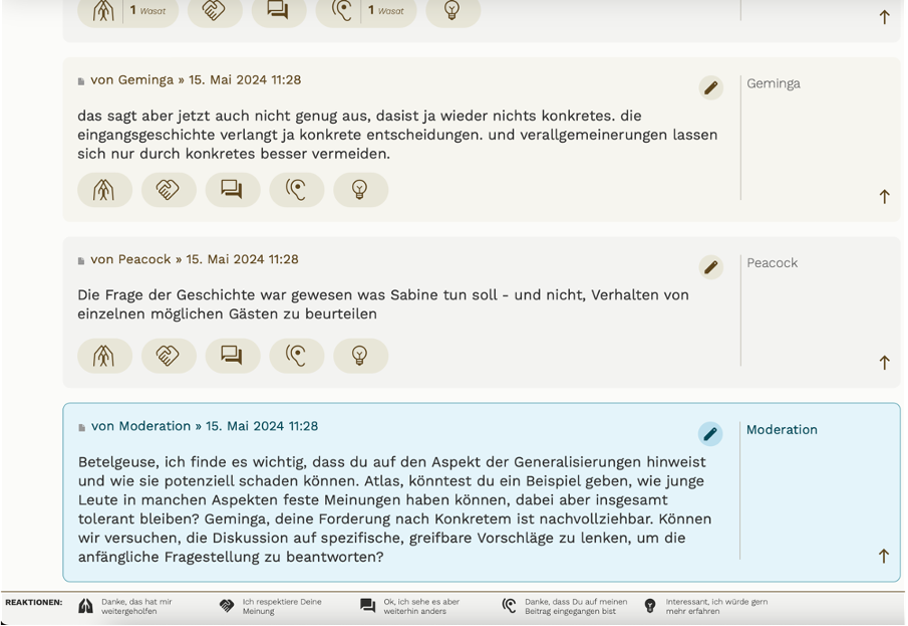
We also trained ChatGPT, a large language model (LLM), to act as a listening-oriented moderator bot. In our experimental online discussions ChatGPT-4 generated moderation interventions in accordance with our 7-page-long moderation guide. The LLM’s task was to intervene when one of the identifiers of bad listening manifested itself: the discussion strayed away from the topic, came to a standstill, became too tense, or demonstrated a lack of substantive engagement, perspective-taking, or reciprocity.
We then conducted 40 half-hour online discussions contrasting 10 groups that received the reaction buttons only but no listening-oriented moderation, 10 groups with buttons plus the human moderation, 10 groups with buttons and the LLM-generated moderation, and 10 control groups that received none of these features. Basic content moderation which removed illegal content, hate speech, etc. without a written intervention was present in all groups. A total of 760 discussants produced 4,466 comments altogether and clicked reaction buttons 5,183 times.
We found that provision of the listening-oriented reaction buttons alone did not significantly enhance the listening exhibited in a discussion. But both human and LLM-generated discussion moderation increased the share of comments that contain listening from roughly a fifth to more than a quarter of all posts.
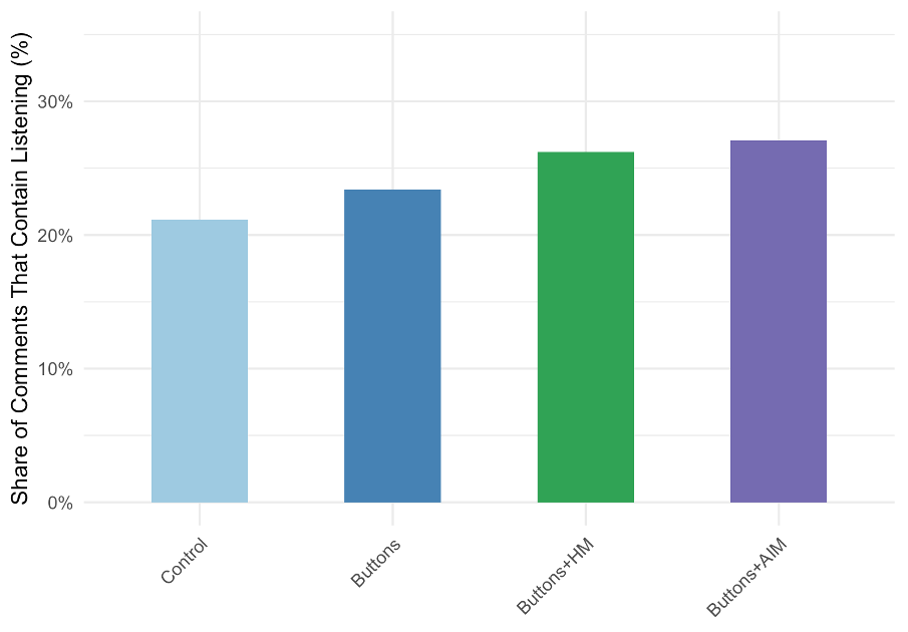
Human and LLM-generated discussion moderation proved equally effective in engendering democratic listening in comments. They also led to very similar perceptions of the overall discussion quality. Discussants in both the human-moderated and LLM-moderated groups equally felt that they could (a) convey their own opinions freely, that they were (b) heard and understood, that discussions (c) helped participants to listen to each other, and that they (d) treated others with respect. However, discussants in human-moderated groups were somewhat more satisfied with the moderation than those subjected to AI moderation.
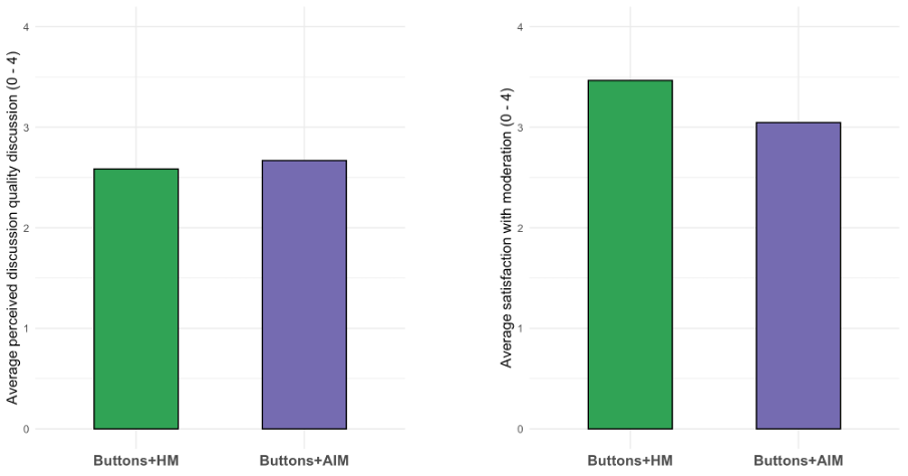
In sum, our experiment proved that interactive discussion moderation can help boost democratic listening in online discussions. AI-generated moderation is equally effective in objectively enhancing listening behavior and creating subjective perceptions of discussion quality. By contrast, AI-generated moderation interventions themselves felt a little less appropriate and helpful to discussants than interventions by our team of human moderators.
Therefore, AI-powered listening-oriented discussion moderation such as the one we developed in this project holds great promise for managing online discussions—be it on news media websites, social media apps or even in dedicated discussion spaces sponsored by civic associations or companies. To realize the democratic listening potential, however, more targeted research efforts are needed to develop adequately functioning AI applications that feel natural to participants. Our project provides the groundwork for such endeavors.